An appeal decision for European case T 0509/18 addresses the question of what the EPO considers to be an enabling disclosure in the field of machine learning. The appellant was TK Holdings Inc. and the application relates to a system and method for determining driver alertness. The Board of Appeal ultimately upheld the appealed decision refusing the application for an insufficient disclosure. The decision underlines that the risk of not disclosing enough details in your application applies equally to machine learning inventions and reinforce a serious lesson for applicants: fail to provide a sufficiently detailed disclosure and your application may be incurably doomed.
The application
TK Holdings Inc filed a patent application for a driver alertness warning system in 2012. It describes how an image is taken of the area where a driver’s head would be. That image is processed to determine the positions of the driver’s head and eyes. Based on those positions, it is determined whether the driver is in an alert state or in a non-alert state, with a warning being issued to the driver when they are determined to be in a non-alert state.
Throughout examination, the EPO held that the method of determining alertness from the positions of the driver’s head and eyes was not clearly defined in the claims and therefore could not contribute to novelty over the prior art. In the appeal, the appellant submitted various claim sets, along with documents relating to the common general knowledge of the skilled person. They argued that “the skilled person would understand the intention of independent claim 1 to carry out a training process in which the driver’s head position and eye vector is registered for predetermined items in the vehicle, and a matrix of inter-point metrics as defined are saved in a look-up-table”.
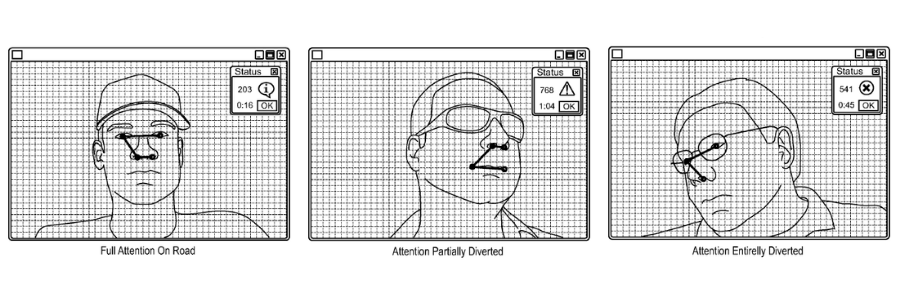
The amendments made in the claim set were based on examples of geometric relationships between facial structures when the driver is in a variety of head and eye positions (shown in the Figure above, taken from the application). These geometric relationships may be referred to as inter-point metrics. The application states that the classification of the driver’s attention state may be derived from the detected silhouette, facial control point, and inter-point metrics, however it does not give any specific examples of how this can be done in practice.
The description discloses a classification training process that is used to register a driver’s head position and eye vector when they are looking at a variety of points within a specific vehicle, giving a number of examples of what these points might be, including A-pillars, the instrument panel, outside mirrors, the rear-view mirror, the passenger floor, and the centre console, among others. A matrix of inter-point metrics is saved and used for a look-up-table classification of the driver’s attention state. There is no further detail in the application on how exactly the look-up-table is achieved from the matrix of inter-point metrics.
The Board’s decision
The result of the appeal was that the Board found that the description of the classification system in the application was insufficient for a skilled person to be able to follow and implement it. The Board took issue with the features “classification training process”, “matrix of inter-point metrics” and “eye vector”, ruling them not to be sufficiently clearly and completely disclosed in the application to enable the skilled person to put them into effect.
According to the Board, the application lacks a disclosure of how to derive, from the matrix of inter-point metrics, a look-up-table classification of the driver’s attention state to be used in deciding on the driver’s attention state. Since a matrix of inter-point metrics is a mathematical object that represents geometric relationships, a specific mathematical method and corresponding criteria would have to be determined. Without them, the skilled person would not know how to construct the look-up-table and therefore would not know how to decide on the driver’s attention state at a given moment based on the video camera image of the driver’s head and eyes. The Board further found that there is no method of criteria provided that would allow the information extracted from the image to be compared with information in the look-up-table. In short, the application failed to disclose how a classification of the attention state is defined or what the training process for deriving it may be, nor how the look-up table is to be used to obtain an attention state from the extracted information.
Ultimately, in spite of the various claim sets presented to the Board by the appellant, since the disclosure of the application lacked fundamental information that would be needed to implement the claimed invention, there was no amendment that the appellant could have made to overcome these issues. Further, the Board did not believe that the general knowledge of the skilled person would be enough for them to implement the invention without undue burden in view of the missing information. The appeal was dismissed.
Analysis of the decision
Sufficiency of disclosure requires that the skilled person can practice the invention using what is disclosed in the application and their common general knowledge, so that the public gets access to the invention in return for granting a temporary monopoly to the patentee. The EPO Guidelines for Examination state that the Examiner must have “serious doubts, substantiated by verifiable facts” in order to raise a sufficiency objection at the EPO. Objections related to sufficiency therefore tend to be rare during examination. This is particularly true of applications related to computing and electronics inventions. However, while there are some particular challenges in disclosing some of the complexities related to machine learning techniques. Deciding on how much should be disclosed can be a tricky judgement call but given the serious consequences of an insufficient disclosure, it is best practice to err on the side of disclosing more rather than less in the application as filed. As this decision demonstrates, at least the basic details of how different instances of data are related and processed to produce the claimed result should always be included.
Prior to this Board of Appeal decision, the EPO had not commented on sufficiency in relation to AI or machine learning applications and this decision is therefore a useful, if not surprising, illustration of the EPO’s approach. Sufficiency of disclosure in the context of AI is a question also being actively considered all major patent jurisdictions. For example, the US Patent and Trademark Office released a set of questions in a public consultation in December 2019 to guide its policy considerations. In October 2020, the results of this public consultation were published, revealing that the majority of commenters believed that there are no unique disclosure considerations for AI inventions. The World Intellectual Property Organization has also conducted a public consultation with a similar scope, the results of which will be made publicly available in due course, so watch this space.
Being quick to the game on this question, the Japanese Patent Office has produced a number of examples of AI and machine learning cases, along with comments on whether there is sufficient disclosure. One of these examples relates to a driver monitoring device in an autonomous vehicle, which may help to shed some light on this Board of Appeal decision at the EPO. The JPO’s example involves obtaining an image of a driver, obtaining a reaction capability score from the image, and switching to manual operation mode when necessary.
In the same way as the EPO decision highlights the lack of detail on how to construct a look-up-table and how an image should be compared with that look-up-table, the JPO example comments on the detail given in the description on how the reaction capability score is assigned to an image. The notes refer to the fact that the hypothetical description discloses using a quick reaction capability score based on numeric parameters that have manually been assigned to the taken images. The description also gives a number of examples of how a driver’s behaviour in an image correlates with the numeric parameter assigned to that image. The JPO believes that, based on common general knowledge, it would therefore be clear to the skilled person that a learning model could be generated that estimates an output in response to an input thorough machine learning with training data containing input data and output data having a correlation.
Although it is hard to know exactly what level of disclosure would have satisfied the EPO’s requirements, we can look to the JPO’s examples as a rough guideline. Perhaps the presence of examples showing how the inter-point metrics relate to the driver’s head position and eye vector and how, in turn, that relates to driver alertness would have helped the TK Holdings application to give enough information for the skilled person to construct a look-up-table. Similarly, had the application given examples of how the head position and eye vector extracted from an image can be compared to the matrix of inter-point metrics in the look-up-table, that might have gone some way towards addressing the EPO’s objections.
As machine learning is a relatively new field in terms of patenting, the full picture on what the EPO considers to be sufficient disclosure in this technical area is still emerging. Hopefully, a relatively consistent picture will emerge on what is considered sufficient disclosure across all jurisdictions.
Key take away
The key practice point to take away from this is that insufficient disclosure in your application may result in the application being incurably flawed as there is no way to make an amendment to ‘fill in’ any missing information. Particularly when drafting machine learning applications, it is important not to make too many sweeping assumptions about the skilled person’s breadth of knowledge but to err on the side of disclosing more rather than less.
Please contact Sinéad, Alexander or your usual Kilburn & Strode advisor if you have any questions.